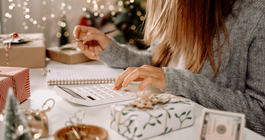
February 27, 2016
Moral victories mean nothing in sports. We hear the same clichés from coaches all the time: “a win is a win” and “a loss is a loss.” Though the focus of a team’s performance (and often the fate of its head coach) relies on the number in the win column, this is not always an accurate representation of the team’s true ability. Different statistical methods can be used to assess the quality of play, putting less weight on the winning percentage and more emphasis on the overall body of work.
Has a team over- or under-performed this season? Is a team currently on a hot or a cold streak? What should we expect from this team for the rest of the year? Was there a turning point (good or bad) in a team’s season? The following statistical model is an attempt to provide insight into these questions and many more.
Back on Jan. 30, the 76ers surprised everyone by playing the then 42-4 Golden State Warriors down to the wire before falling on a Harrison Barnes three pointer at the buzzer. While that loss counts the same as every other loss in the standings, in a statistical model, the 76ers are actually rewarded for their performance. While they were heavy underdogs to the defending champions, falling by only three points was an indication that the 76ers are a significantly better team than their record indicated.
However, point differential can be deceiving. An 85-75 defensive battle where points are difficult to come by is not nearly as close as a high scoring 132-122 game even though the margin of victory is 10 points in each. Instead, our statistical model uses the difference in the percentage of points scored as a measure of how well each team performed.
For example, consider an NBA game with a final score of 110-90. The winning team would have scored 55 percent of the points compared to 45 percent scored by the losing team. Rather than use the 20-point win as a measure of how well the home team played, we use the 10 percent advantage it had in the percentage of points scored to accommodate for teams that play solid defense.
A team that wins many close games may have a slightly inflated record; however, this will not fool the model. That team will be perceived as being worse than a team with the same record that has lost many close games instead.
These differences in the percentage of points scored can be used to calculate the overall rating of a team’s seasonal performance using an analysis of variance (ANOVA) model. These ratings are then standardized to provide a measure of how much better or worse a team is from the league average.
A team with a rating of zero would be considered a perfectly average team. Approximately two-thirds of teams will have ratings between -1 and 1, while about 95 percent will be rated between -2 and 2. Only the best teams of all time will exceed a rating of 3, while only the worst will approach -3. These ratings can be thought of as the strength and depth of a team on paper.
Our model also includes a weighted component that takes into consideration how a team has performed recently. Since teams tend to go on hot and cold streaks, our perception of how well they will play in an upcoming game also incorporates their recent performance, rewarding teams for going on winning streaks and punishing them for losing streaks.
However, the most interesting facet of the model is the ability to simulate entire seasons to answer the questions we posed at the beginning of the article. Logistic regression is yet another statistical technique that allows to predict the probability or chance that an event occurs. We use the difference between the weighted ratings of two teams to calculate the probability of a win.
Both home court advantage and the parity of the league are taken into consideration when calculating these probabilities. Since upsets are not as prevalent, the difference between the ratings of two teams plays a much larger role in the NBA than in the NHL, where few games can be considered surprising upsets.
Though this article has focused on the relevance of the model in the NBA, it can and has been applied to all four major sports. Through these simulations, we can provide midseason projections as to how a team may finish, determine how lucky a team was during a season, identify potential turning points and answer many other enigmatic questions.