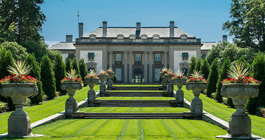
February 25, 2016
Computer programs designed to assist judges during criminal arraignments could cut the number of repeat domestic violence arrests in half, according to a study from the University of Pennsylvania.
When a suspect is first arrested and brought before a judge, that magistrate must decide if the suspect will be released on bail. The study authors say that the statistical program they tested could help judges make better predictions of who is truly a risk.
"It's much more accurate than current practice," said criminology professor Richard Berk, who co-wrote the study with Susan Sorenson and Geoffrey Barnes. "It's not perfect — it makes mistakes — but the baseline is current practice."
The program was not used for any real-life court decisions, but it was based on real-life data: the records of more than 28,000 domestic violence arraignments over four years in an unnamed city.
Researchers put in all the information they could find on each suspect's criminal history, plus their age, gender and whether they lived in a high-crime ZIP code. Using that data, the machine "learned" how to predict which suspects were most likely to get arrested on domestic violence charges again within two years of their first arrest.
Predictions that someone would not be arrested for domestic violence again were accurate 89 percent of the time. That means that if a judge relied on this program, out of every 100 people released, only 11 would get arrested again.
This is almost half the current re-arrest rate for domestic violence charges, which is around 20 percent.
"I was surprised by how much better we're able to predict those individuals who are going to commit new domestic violence crimes and how much it approves the ability to reduce domestic violence," said Berk.
However, predictions that someone would be a re-offender were not nearly as accurate as predictions that someone would not be a re-offender. This was on purpose.
The algorithm was designed so it would be more likely to produce "false positives" (a low-risk person is labeled high-risk) than "false negatives" (vice-versa). The priority was to minimize the risk that the algorithm would allow a dangerous offender to return to the streets and commit another act of domestic violence.
"It's very, very costly to release somebody who's going to commit a violent crime...It didn't take a whole lot of evidence to determine that somebody was high-risk, because you really don't want to make that mistake," said Berk.
When asked if this would increase the number of people kept in jail after arrests, Berk said that the program would have the exact opposite effect.
"Fewer, actually, because we're also not going to keep people who don't belong there. We're going to make fewer mistakes of both kinds," he said.
The paper also notes that judges do not have to keep a person labeled as high-risk in jail but could instead turn to intervention programs or probation with home visits. They could also devote more time to examining the borderline cases closely and then release even more people.
Moreover, the authors emphasize that the computer program would merely be a tool, not the final decision-maker.
"It's just a piece of information. The judges still have discretion. They do what they think is best," said Berk.
He hopes to continue his research into how machine learning can predict other kinds of crimes, like drug or weapons offenses. Eventually, the criminal justice system may rely on machines to make decisions with mathematical precision.
Read the full study here.